From close-listening to distant-listening: Developing tools for Speech-Music discrimination of Danish music radio
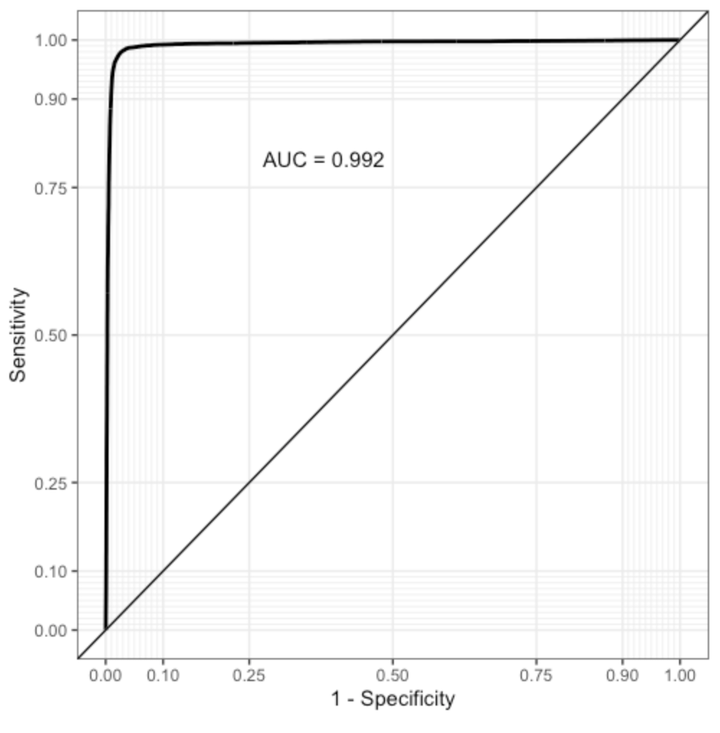
Abstract
Digitization has changed flow music radio. Competition from music streaming services like Spotify and iTunes has to a large extend outperformed traditional playlist radio, and the global dissemination of software generated playlists in public service radio stations in the 1990s has superseded the passionate music radio host. But digitization has also changed the way we can do research in radio. In Denmark digitization of almost all radio programming back to 1989, have made it possible to actually listen to the archive to investigate how radio content has changed historically. This article investigates the research question: How has the distribution of music and talk on the Danish Broadcasting Corporation’s radio channel P3 developed 1989-2019 by comparing a qualitative case study with a new large-scale study. Methodologically this shift from a close listening to a few programs to large-scale distant listening to more than 65,000 hours of radio enables us to discuss and critically compare the methods, results, strengths and shortcomings of the two analysis. Previous studies have demonstrated that Convolutional Neural Networks (CNNs) trained for image recognition of spectograms of the audio outperforms alternative approaches, such as Support Vector Machines (SVMs). The large-scale study presented shows that the CNN-based approach generalizes well, even without fine-tuning, to speech and music classification in Danish radio, with an overall accuracy of 98%.
Twitter Thread
Want to know how digitization and institutionalization have changed the flow of music? How to go from close listening to distant listening?, or are you just interested in State-of-the-Art performance in Danish music and speech segmentation? Read further! 1/🧵 pic.twitter.com/jN98LF0mHS
— Kenneth Enevoldsen (@KCEnevoldsen) March 15, 2021
In our recent paper (https://t.co/keekDAM3SM) @ibenhave and I examine the effect of music streaming services, standardization, and institutional changes to the relationship between speech and music in Danish National Radio. Using both close and distant listening 2/🧵
— Kenneth Enevoldsen (@KCEnevoldsen) March 15, 2021
For distant listening we use machine learning techniques (CNN) to obtain an accuracy of 98% on speech music segmentation! Even including ambiguous categories such as jingles, silence and a mixture of speech and music only decrease performance marginally to 96%! 3/🧵 pic.twitter.com/MEpDukqtgy
— Kenneth Enevoldsen (@KCEnevoldsen) March 15, 2021
Furthermore, we provide a comparison of machine learning techniques for speech music segmentation and show that these perform well despite never being trained on Danish specifically. Furthermore, we show that the CNN's applied to spectrograms clearly outperform SVM. 4/🧵 pic.twitter.com/1IFH7h3WIb
— Kenneth Enevoldsen (@KCEnevoldsen) March 15, 2021
Using both close and distant listening we provide a more saturated picture of the development of Danish Radio and show that increased competition and the introduction of standardized formats meaningfully change the relationship between music and speech. 5/🧵 pic.twitter.com/eM2FGLdUQt
— Kenneth Enevoldsen (@KCEnevoldsen) March 15, 2021
🔗 links:https://t.co/keekDAM3SM
— Kenneth Enevoldsen (@KCEnevoldsen) March 15, 2021
Be sure to also check of the original implementation of the CNN by @michpapakos:
🖥️ GitHub: https://t.co/cIHXA9JSmg
And naturally many thanks to @ibenhave and @DetKglBibliotek for a wonderful collaboration.
— Kenneth Enevoldsen (@KCEnevoldsen) March 15, 2021